Note
Go to the end to download the full example code or to run this example in your browser via Binder
Eddy detection : Antartic Circumpolar Current¶
This script detect eddies on the ADT field, and compute u,v with the method add_uv (use it only if the Equator is avoided)
Two detections are provided : with a filtered ADT and without filtering
from datetime import datetime
from matplotlib import pyplot as plt, style
from py_eddy_tracker import data
from py_eddy_tracker.dataset.grid import RegularGridDataset
pos_cb = [0.1, 0.52, 0.83, 0.015]
pos_cb2 = [0.1, 0.07, 0.4, 0.015]
def quad_axes(title):
style.use("default")
fig = plt.figure(figsize=(13, 10))
fig.suptitle(title, weight="bold", fontsize=14)
axes = list()
ax_pos = dict(
topleft=[0.1, 0.54, 0.4, 0.38],
topright=[0.53, 0.54, 0.4, 0.38],
botleft=[0.1, 0.09, 0.4, 0.38],
botright=[0.53, 0.09, 0.4, 0.38],
)
for key, position in ax_pos.items():
ax = fig.add_axes(position)
ax.set_xlim(5, 45), ax.set_ylim(-60, -37)
ax.set_aspect("equal"), ax.grid(True)
axes.append(ax)
if "right" in key:
ax.set_yticklabels("")
return fig, axes
def set_fancy_labels(fig, ticklabelsize=14, labelsize=14, labelweight="semibold"):
for ax in fig.get_axes():
ax.grid()
ax.grid(which="major", linestyle="-", linewidth="0.5", color="black")
if ax.get_ylabel() != "":
ax.set_ylabel(ax.get_ylabel(), fontsize=labelsize, fontweight=labelweight)
if ax.get_xlabel() != "":
ax.set_xlabel(ax.get_xlabel(), fontsize=labelsize, fontweight=labelweight)
if ax.get_title() != "":
ax.set_title(ax.get_title(), fontsize=labelsize, fontweight=labelweight)
ax.tick_params(labelsize=ticklabelsize)
Load Input grid, ADT is used to detect eddies
margin = 30
kw_data = dict(
filename=data.get_demo_path("nrt_global_allsat_phy_l4_20190223_20190226.nc"),
x_name="longitude",
y_name="latitude",
# Manual area subset
indexs=dict(
latitude=slice(100 - margin, 220 + margin),
longitude=slice(0, 230 + margin),
),
)
g_raw = RegularGridDataset(**kw_data)
g_raw.add_uv("adt")
g = RegularGridDataset(**kw_data)
g.copy("adt", "adt_low")
g.bessel_high_filter("adt", 700)
g.bessel_low_filter("adt_low", 700)
g.add_uv("adt")
Identification¶
Run the identification step with slices of 2 mm
/home/docs/checkouts/readthedocs.org/user_builds/py-eddy-tracker/conda/latest/lib/python3.10/site-packages/numpy-1.24.3-py3.10-linux-x86_64.egg/numpy/lib/function_base.py:4737: UserWarning: Warning: 'partition' will ignore the 'mask' of the MaskedArray.
arr.partition(
Figures¶
kw_adt = dict(vmin=-1.5, vmax=1.5, cmap=plt.get_cmap("RdBu_r", 30))
fig, axs = quad_axes("General properties field")
g_raw.display(axs[0], "adt", **kw_adt)
axs[0].set_title("Total ADT (m)")
m = g.display(axs[1], "adt_low", **kw_adt)
axs[1].set_title("ADT (m) large scale, cutoff at 700 km")
m2 = g.display(axs[2], "adt", cmap=plt.get_cmap("RdBu_r", 20), vmin=-0.5, vmax=0.5)
axs[2].set_title("ADT (m) high-pass filtered, a cutoff at 700 km")
cb = plt.colorbar(m, cax=axs[0].figure.add_axes(pos_cb), orientation="horizontal")
cb.set_label("ADT (m)", labelpad=0)
cb2 = plt.colorbar(m2, cax=axs[2].figure.add_axes(pos_cb2), orientation="horizontal")
cb2.set_label("ADT (m)", labelpad=0)
set_fancy_labels(fig)
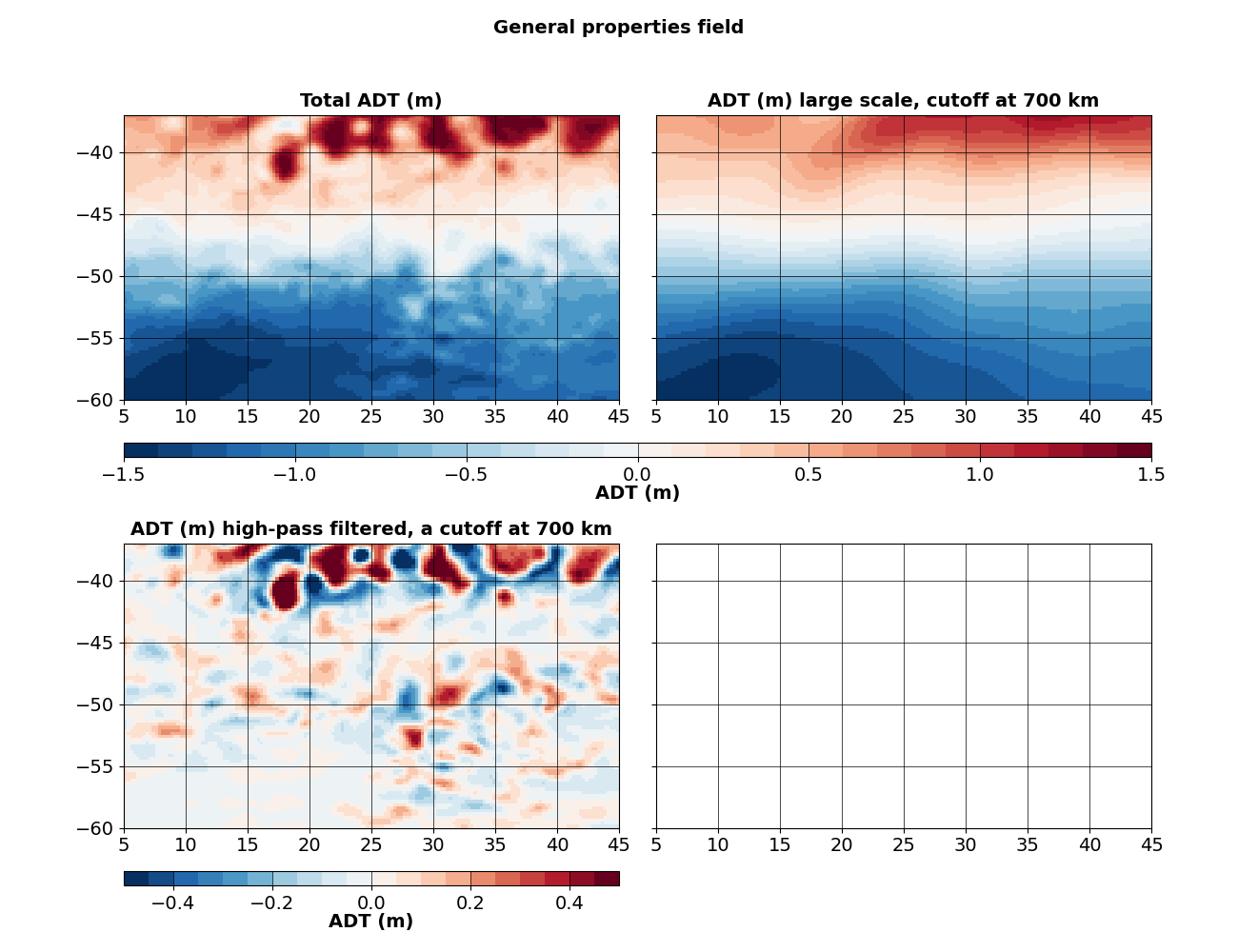
The large-scale North-South gradient is removed by the filtering step.
fig, axs = quad_axes("")
axs[0].set_title("Without filter")
axs[0].set_ylabel("Contours used in eddies")
axs[1].set_title("With filter")
axs[2].set_ylabel("Closed contours but not used")
g_raw.contours.display(axs[0], lw=0.5, only_used=True)
g.contours.display(axs[1], lw=0.5, only_used=True)
g_raw.contours.display(axs[2], lw=0.5, only_unused=True)
g.contours.display(axs[3], lw=0.5, only_unused=True)
set_fancy_labels(fig)
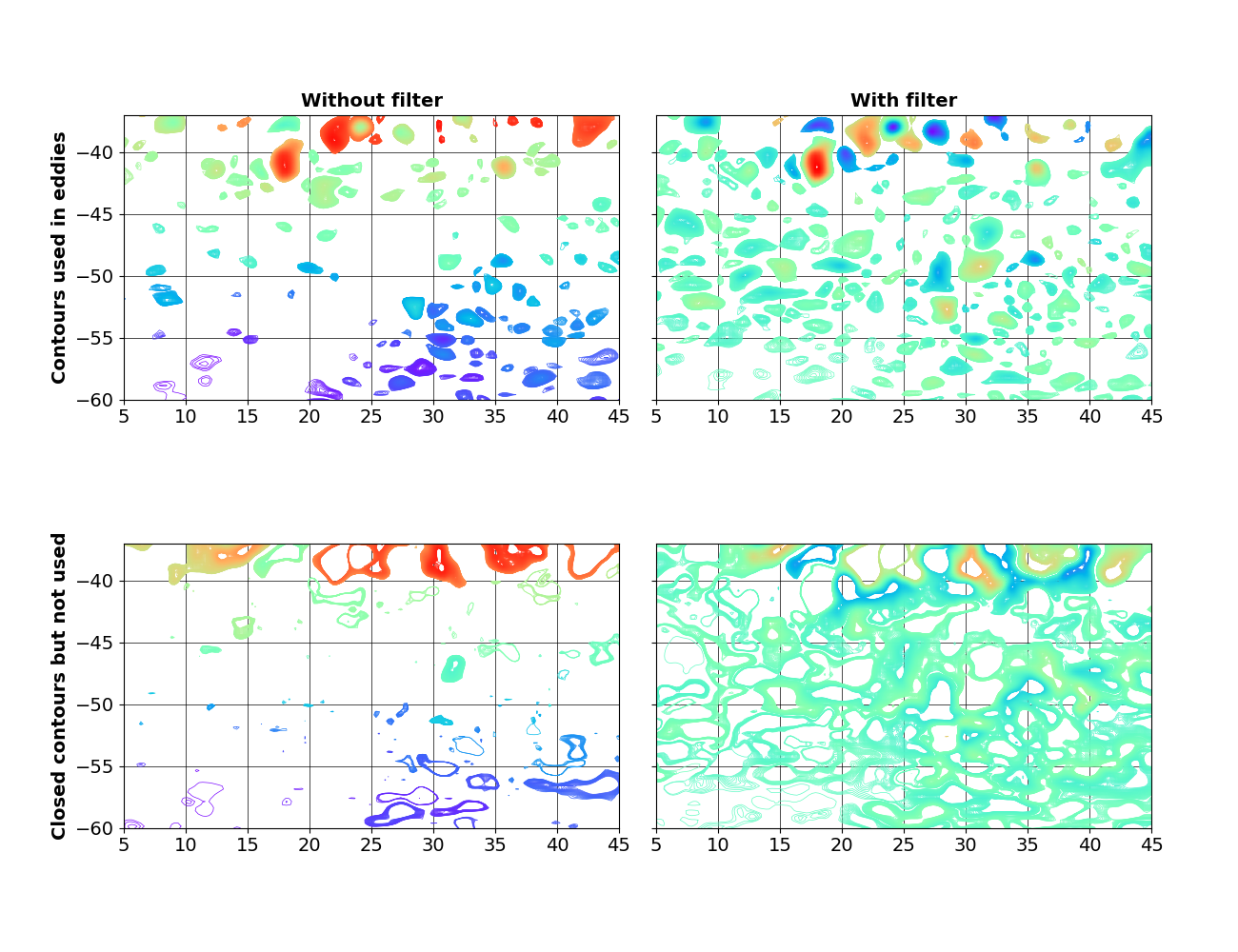
Removing the large-scale North-South gradient reveals closed contours in the South-Western corner of the ewample region.
kw = dict(ref=-10, linewidth=0.75)
kw_a = dict(color="r", label="Anticyclonic ({nb_obs} eddies)")
kw_c = dict(color="b", label="Cyclonic ({nb_obs} eddies)")
kw_filled = dict(vmin=0, vmax=100, cmap="Spectral_r", lut=20, intern=True, factor=100)
fig, axs = quad_axes("Comparison between two detections")
# Match with intern/inner contour
i_a, j_a, s_a = a_.match(a, intern=True, cmin=0.15)
i_c, j_c, s_c = c_.match(c, intern=True, cmin=0.15)
a_.index(i_a).filled(axs[0], s_a, **kw_filled)
a.index(j_a).filled(axs[1], s_a, **kw_filled)
c_.index(i_c).filled(axs[0], s_c, **kw_filled)
m = c.index(j_c).filled(axs[1], s_c, **kw_filled)
cb = plt.colorbar(m, cax=axs[0].figure.add_axes(pos_cb), orientation="horizontal")
cb.set_label("Similarity index (%)", labelpad=-5)
a_.display(axs[0], **kw, **kw_a), c_.display(axs[0], **kw, **kw_c)
a.display(axs[1], **kw, **kw_a), c.display(axs[1], **kw, **kw_c)
axs[0].set_title("Without filter")
axs[0].set_ylabel("Detection")
axs[1].set_title("With filter")
axs[2].set_ylabel("Contours' rejection criteria")
g_raw.contours.display(axs[2], lw=0.5, only_unused=True, display_criterion=True)
g.contours.display(axs[3], lw=0.5, only_unused=True, display_criterion=True)
for ax in axs:
ax.legend()
set_fancy_labels(fig)
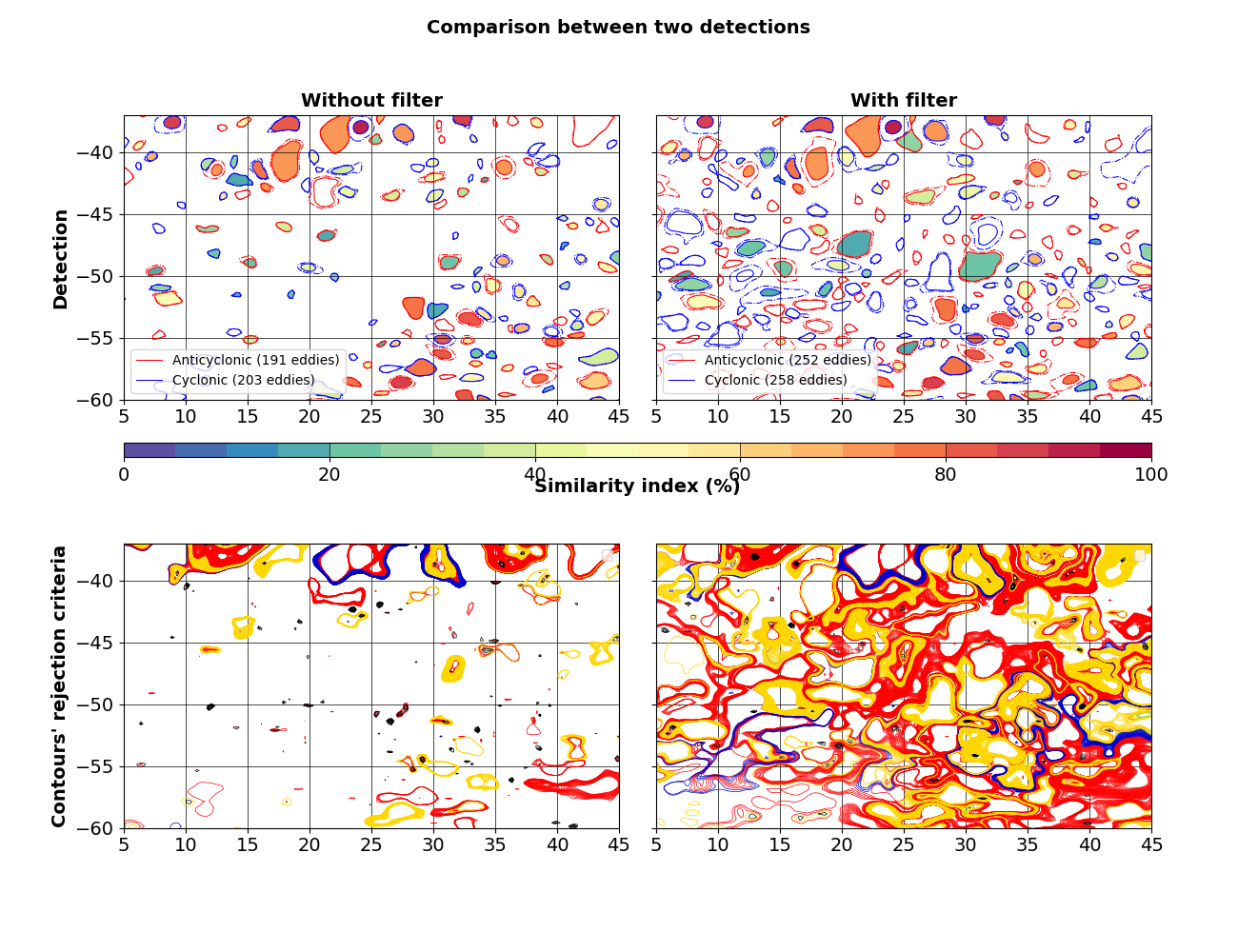
No artists with labels found to put in legend. Note that artists whose label start with an underscore are ignored when legend() is called with no argument.
No artists with labels found to put in legend. Note that artists whose label start with an underscore are ignored when legend() is called with no argument.
Very similar eddies have Similarity Indexes >= 40%
- Criteria for rejecting a contour :
Accepted (green)
Rejection for shape error (red)
Masked value within contour (blue)
Under or over the pixel limit bounds (black)
Amplitude criterion (yellow)
i_a, j_a = i_a[s_a >= 0.4], j_a[s_a >= 0.4]
i_c, j_c = i_c[s_c >= 0.4], j_c[s_c >= 0.4]
fig = plt.figure(figsize=(12, 12))
fig.suptitle(f"Scatter plot (A : {i_a.shape[0]}, C : {i_c.shape[0]} matches)")
for i, (label, field, factor, stop) in enumerate(
(
("Speed radius (km)", "radius_s", 0.001, 120),
("Effective radius (km)", "radius_e", 0.001, 120),
("Amplitude (cm)", "amplitude", 100, 25),
("Speed max (cm/s)", "speed_average", 100, 25),
)
):
ax = fig.add_subplot(2, 2, i + 1, title=label)
ax.set_xlabel("Without filter")
ax.set_ylabel("With filter")
ax.plot(
a_[field][i_a] * factor,
a[field][j_a] * factor,
"r.",
label="Anticyclonic",
)
ax.plot(
c_[field][i_c] * factor,
c[field][j_c] * factor,
"b.",
label="Cyclonic",
)
ax.set_aspect("equal"), ax.grid()
ax.plot((0, 1000), (0, 1000), "g")
ax.set_xlim(0, stop), ax.set_ylim(0, stop)
ax.legend()
set_fancy_labels(fig)
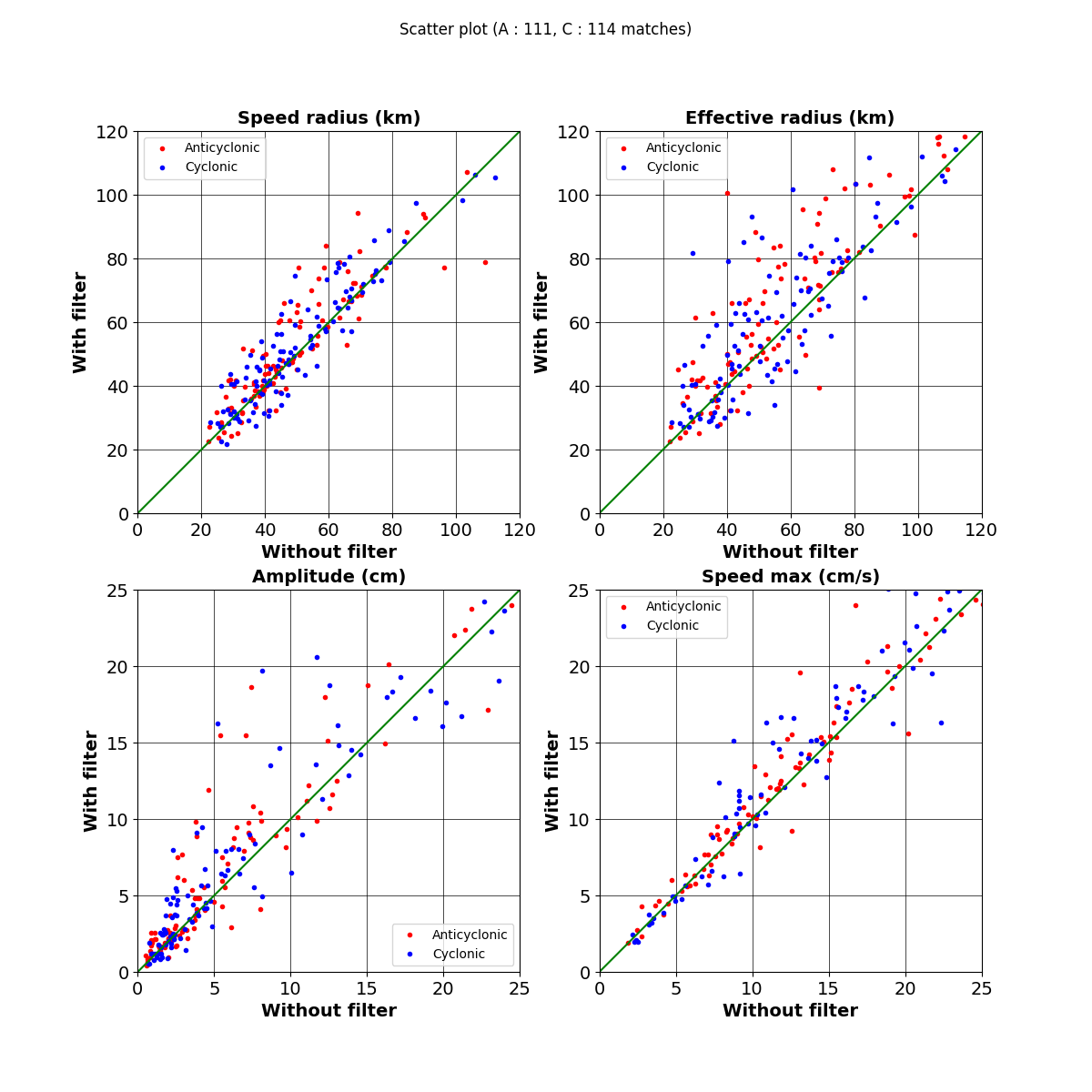
Total running time of the script: ( 0 minutes 21.148 seconds)