Note
Go to the end to download the full example code or to run this example in your browser via Binder
Ioannou case¶
Figure 10 from https://doi.org/10.1002/2017JC013158
We want to find the Ierapetra Eddy described above in a network demonstration run.
import re
from matplotlib import colors, pyplot as plt
from matplotlib.animation import FuncAnimation
from matplotlib.ticker import FuncFormatter
from numpy import arange, array, pi, where
from py_eddy_tracker.appli.gui import Anim
from py_eddy_tracker.data import get_demo_path
from py_eddy_tracker.generic import coordinates_to_local
from py_eddy_tracker.gui import GUI_AXES
from py_eddy_tracker.observations.network import NetworkObservations
from py_eddy_tracker.poly import fit_ellipse
class VideoAnimation(FuncAnimation):
def _repr_html_(self, *args, **kwargs):
"""To get video in html and have a player"""
content = self.to_html5_video()
return re.sub(
r'width="[0-9]*"\sheight="[0-9]*"', 'width="100%" height="100%"', content
)
def save(self, *args, **kwargs):
if args[0].endswith("gif"):
# In this case gif is used to create thumbnail which is not used but consume same time than video
# So we create an empty file, to save time
with open(args[0], "w") as _:
pass
return
return super().save(*args, **kwargs)
@FuncFormatter
def formatter(x, pos):
return (timedelta(x) + datetime(1950, 1, 1)).strftime("%d/%m/%Y")
def start_axes(title=""):
fig = plt.figure(figsize=(13, 6))
ax = fig.add_axes([0.03, 0.03, 0.90, 0.94], projection=GUI_AXES)
ax.set_xlim(19, 29), ax.set_ylim(31, 35.5)
ax.set_aspect("equal")
ax.set_title(title, weight="bold")
return ax
def timeline_axes(title=""):
fig = plt.figure(figsize=(15, 5))
ax = fig.add_axes([0.03, 0.06, 0.90, 0.88])
ax.set_title(title, weight="bold")
ax.xaxis.set_major_formatter(formatter), ax.grid()
return ax
def update_axes(ax, mappable=None):
ax.grid(True)
if mappable:
return plt.colorbar(mappable, cax=ax.figure.add_axes([0.94, 0.05, 0.01, 0.9]))
We know the network ID, we will get directly
ioannou_case = NetworkObservations.load_file(get_demo_path("network_med.nc")).network(
651
)
print(ioannou_case.infos())
11948 obs 244 segments
It seems that this network is huge! Our case is visible at 22E 33.5N
ax = start_axes()
ioannou_case.plot(ax, color_cycle=ioannou_case.COLORS)
update_axes(ax)
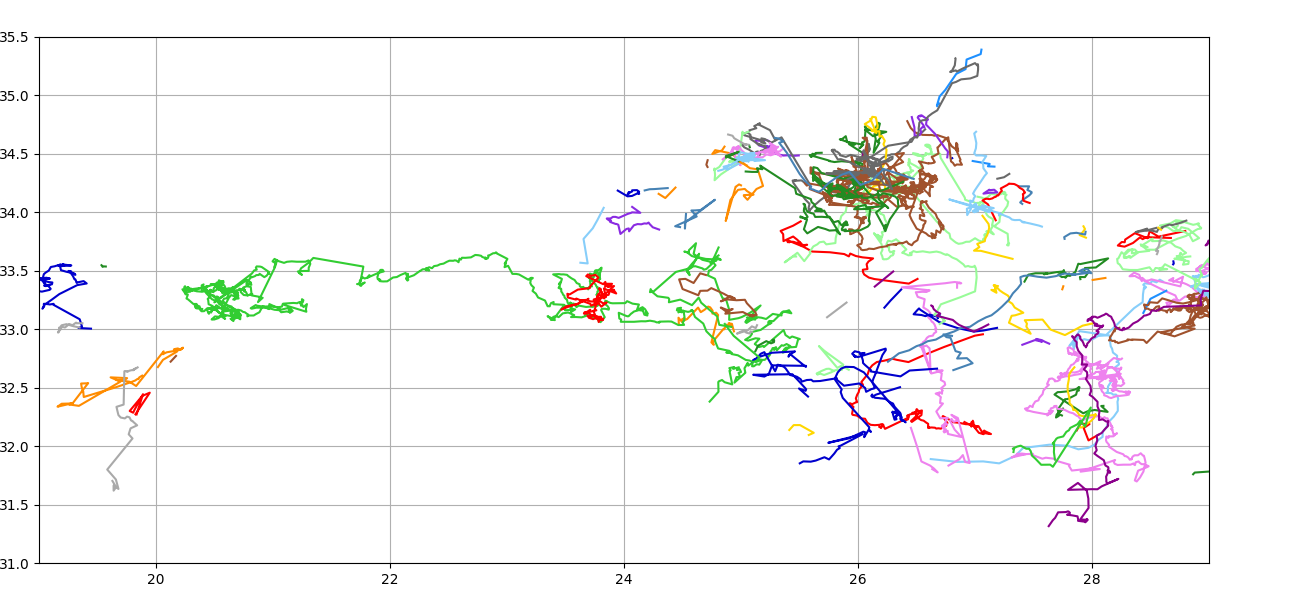
Full Timeline¶
The network span for many years… How to cut the interesting part?
fig = plt.figure(figsize=(15, 5))
ax = fig.add_axes([0.04, 0.05, 0.92, 0.92])
ax.xaxis.set_major_formatter(formatter), ax.grid()
_ = ioannou_case.display_timeline(ax)
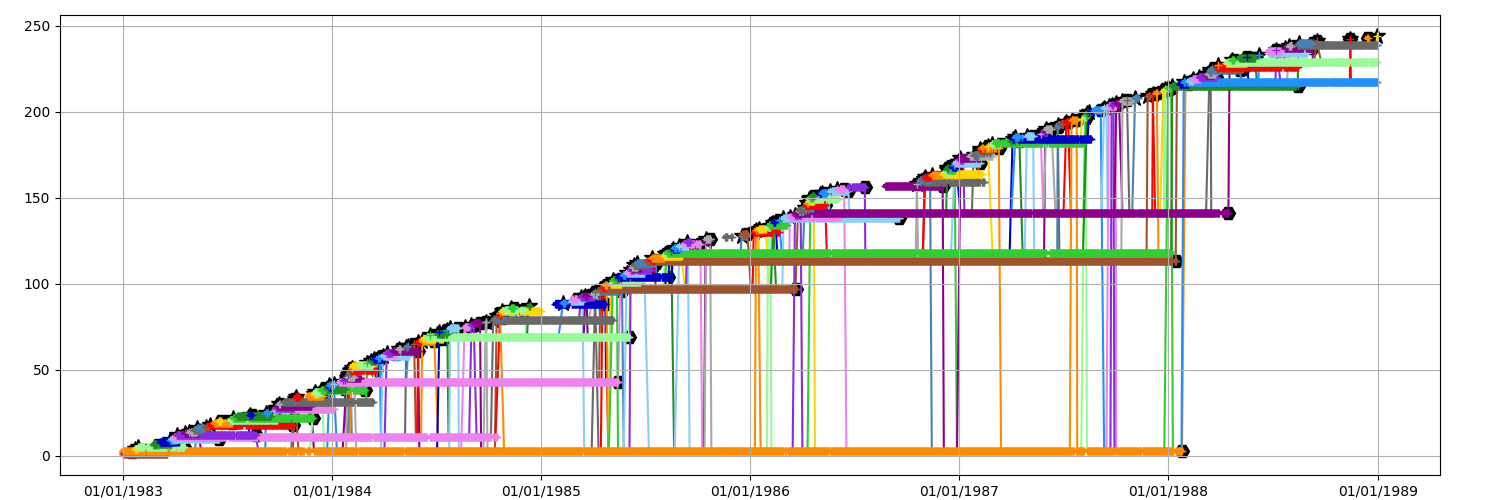
Sub network and new numbering¶
Here we chose to keep only the order 3 segments relatives to our chosen eddy
i = where(
(ioannou_case.lat > 33)
* (ioannou_case.lat < 34)
* (ioannou_case.lon > 22)
* (ioannou_case.lon < 23)
* (ioannou_case.time > 20630)
* (ioannou_case.time < 20650)
)[0][0]
close_to_i3 = ioannou_case.relative(i, order=3)
close_to_i3.numbering_segment()
Anim¶
Quick movie to see better!
a = Anim(
close_to_i3,
figsize=(12, 4),
cmap=colors.ListedColormap(
list(close_to_i3.COLORS), name="from_list", N=close_to_i3.segment.max() + 1
),
nb_step=7,
dpi=70,
field_color="segment",
field_txt="segment",
)
a.ax.set_xlim(19, 30), a.ax.set_ylim(32, 35.25)
a.txt.set_position((21.5, 32.7))
# We display in video only from the 100th day to the 500th
kwargs = dict(frames=arange(*a.period)[100:501], interval=100)
ani = VideoAnimation(a.fig, a.func_animation, **kwargs)
Classic display¶
ax = timeline_axes()
_ = close_to_i3.display_timeline(ax)
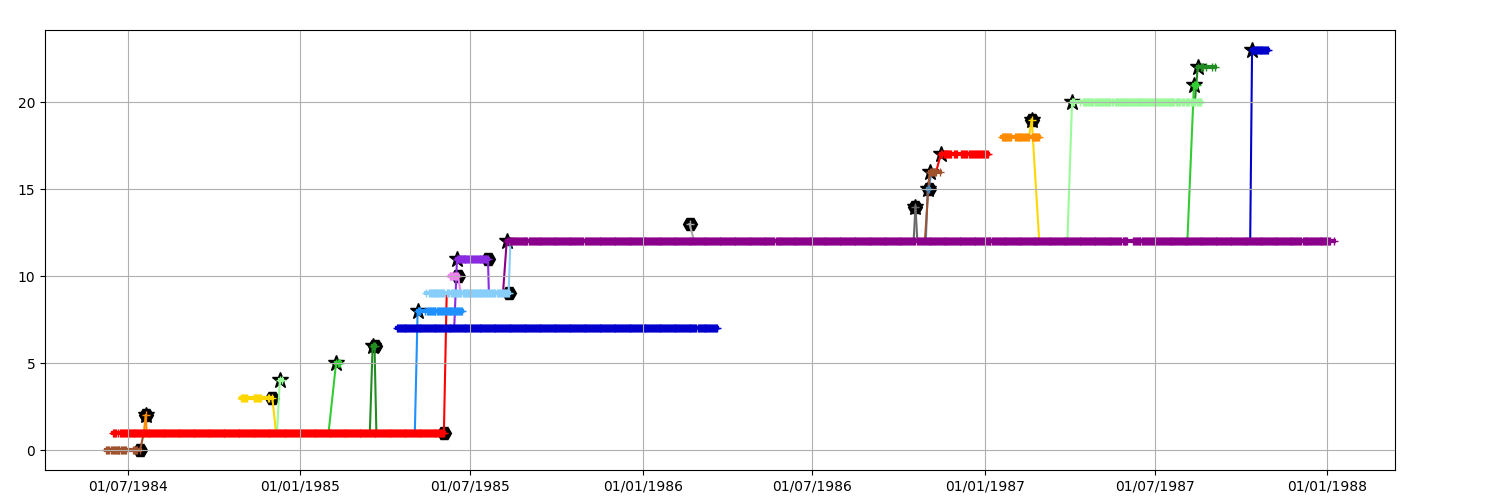
ax = start_axes("")
n_copy = close_to_i3.copy()
n_copy.position_filter(2, 4)
n_copy.plot(ax, color_cycle=n_copy.COLORS)
update_axes(ax)
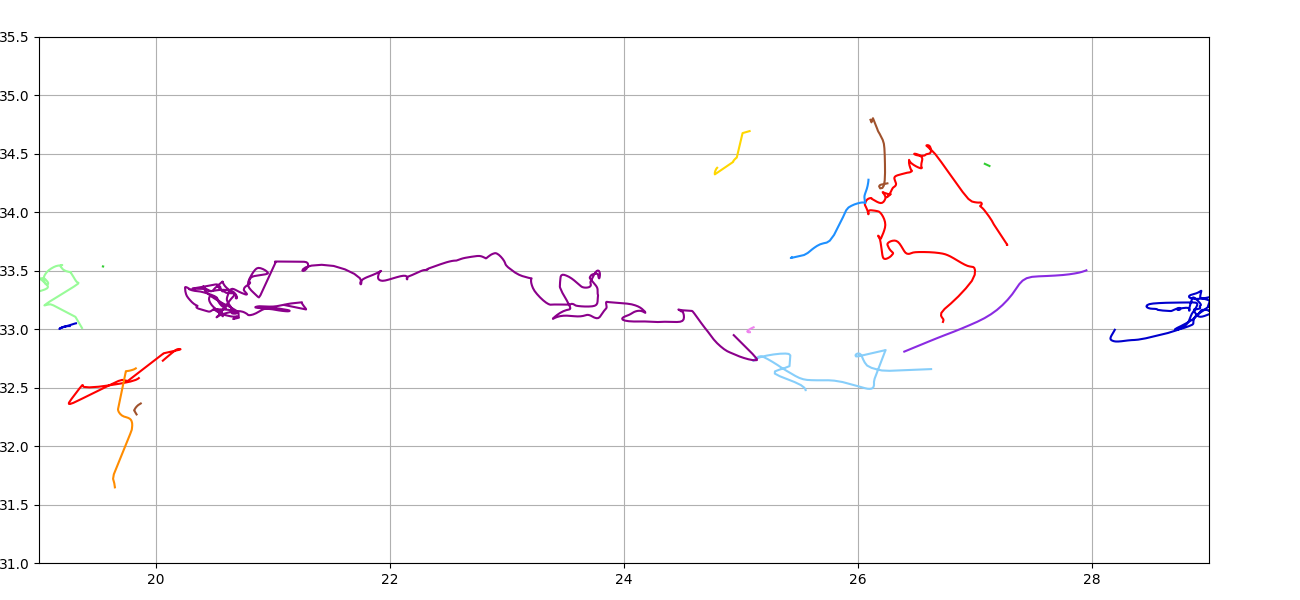
Latitude Timeline¶
ax = timeline_axes(f"Close segments ({close_to_i3.infos()})")
n_copy = close_to_i3.copy()
n_copy.median_filter(15, "time", "latitude")
_ = n_copy.display_timeline(ax, field="lat", method="all")
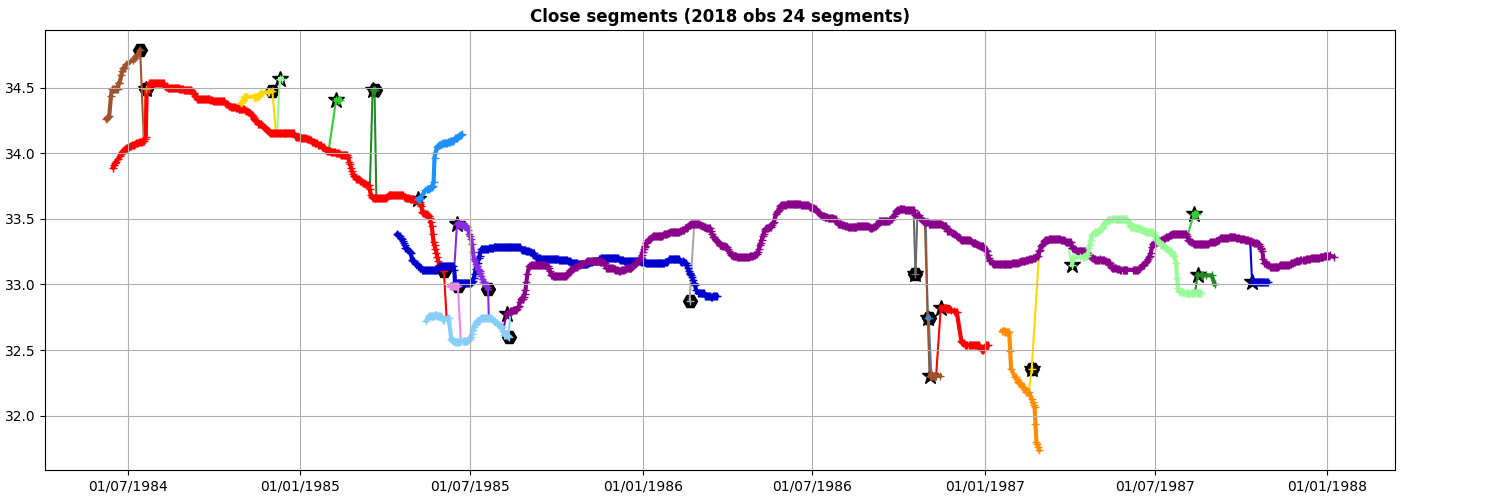
Local radius timeline¶
Effective (bold) and Speed (thin) Radius together
n_copy.median_filter(2, "time", "radius_e")
n_copy.median_filter(2, "time", "radius_s")
for b0, b1 in [
(datetime(i, 1, 1), datetime(i, 12, 31)) for i in (2004, 2005, 2006, 2007)
]:
ref, delta = datetime(1950, 1, 1), 20
b0_, b1_ = (b0 - ref).days, (b1 - ref).days
ax = timeline_axes()
ax.set_xlim(b0_ - delta, b1_ + delta)
ax.set_ylim(10, 115)
ax.axvline(b0_, color="k", lw=1.5, ls="--"), ax.axvline(
b1_, color="k", lw=1.5, ls="--"
)
n_copy.display_timeline(
ax, field="radius_e", method="all", lw=4, markersize=8, factor=1e-3
)
n_copy.display_timeline(
ax, field="radius_s", method="all", lw=1, markersize=3, factor=1e-3
)
Parameters timeline¶
Effective Radius
kw = dict(s=35, cmap=plt.get_cmap("Spectral_r", 8), zorder=10)
ax = timeline_axes()
m = close_to_i3.scatter_timeline(ax, "radius_e", factor=1e-3, vmin=20, vmax=100, **kw)
cb = update_axes(ax, m["scatter"])
cb.set_label("Effective radius (km)")
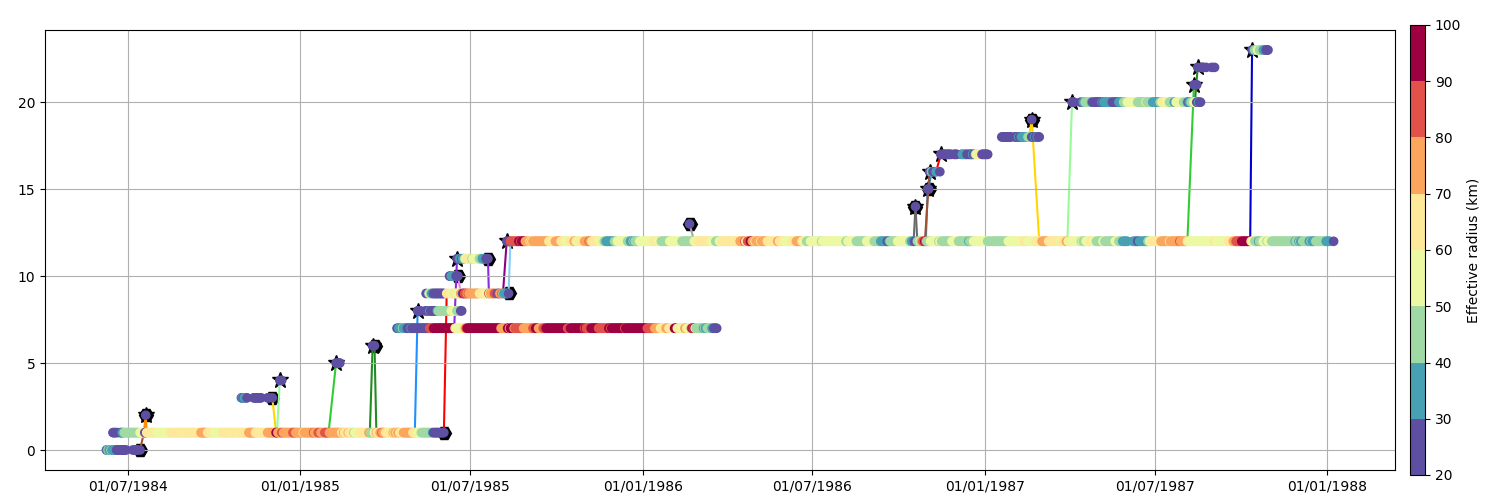
Shape error
ax = timeline_axes()
m = close_to_i3.scatter_timeline(ax, "shape_error_e", vmin=14, vmax=70, **kw)
cb = update_axes(ax, m["scatter"])
cb.set_label("Effective shape error")
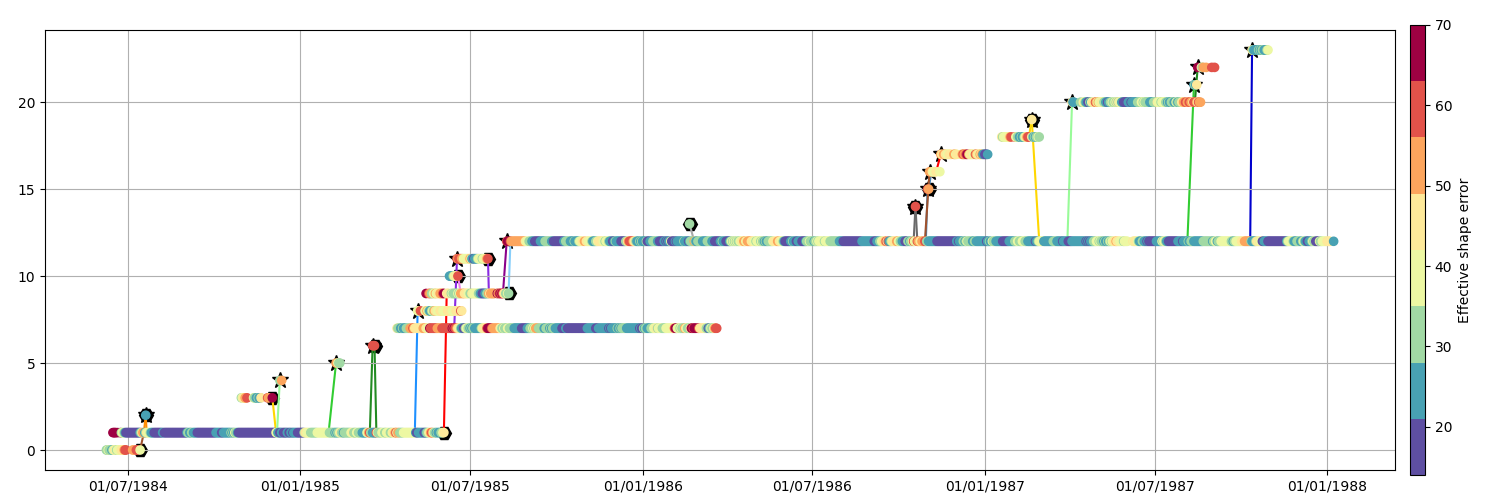
Rotation angle¶
For each obs, fit an ellipse to the contour, with theta the angle from the x-axis, a the semi ax in x direction and b the semi ax in y dimension
theta_ = list()
a_ = list()
b_ = list()
for obs in close_to_i3:
x, y = obs["contour_lon_s"], obs["contour_lat_s"]
x0_, y0_ = x.mean(), y.mean()
x_, y_ = coordinates_to_local(x, y, x0_, y0_)
x0, y0, a, b, theta = fit_ellipse(x_, y_)
theta_.append(theta)
a_.append(a)
b_.append(b)
a_ = array(a_)
b_ = array(b_)
Theta
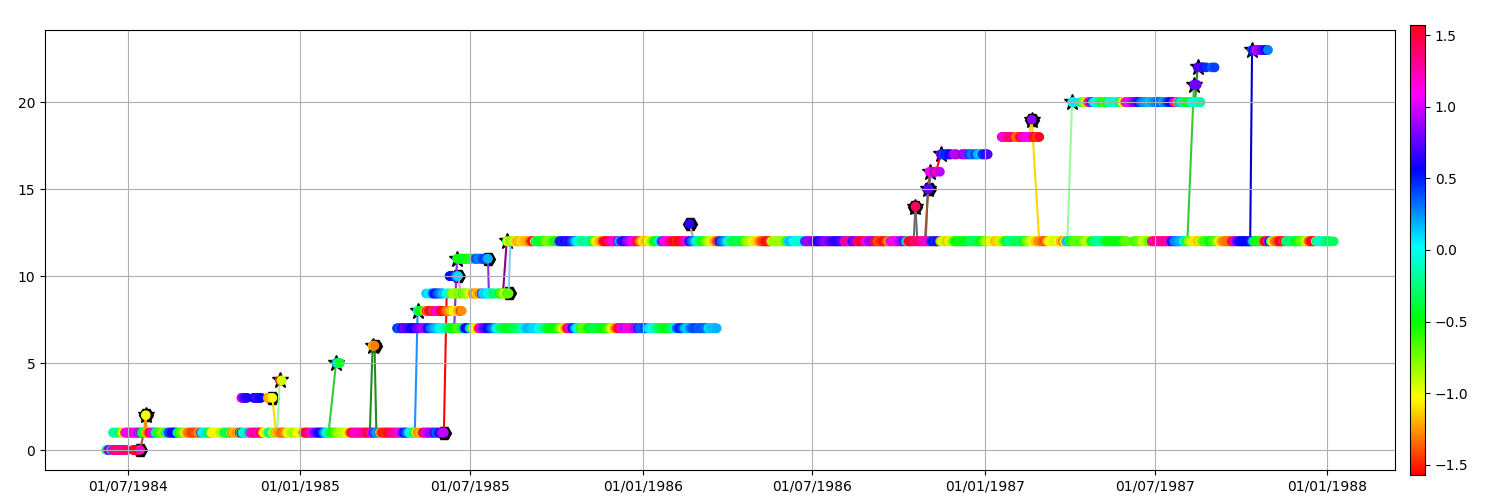
a
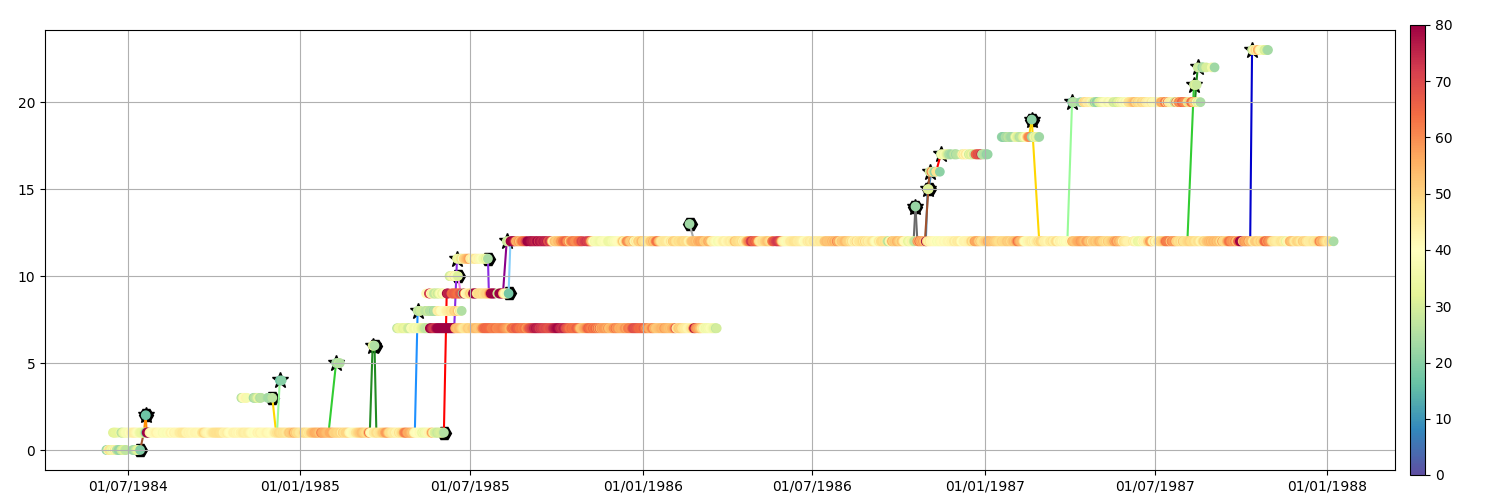
b
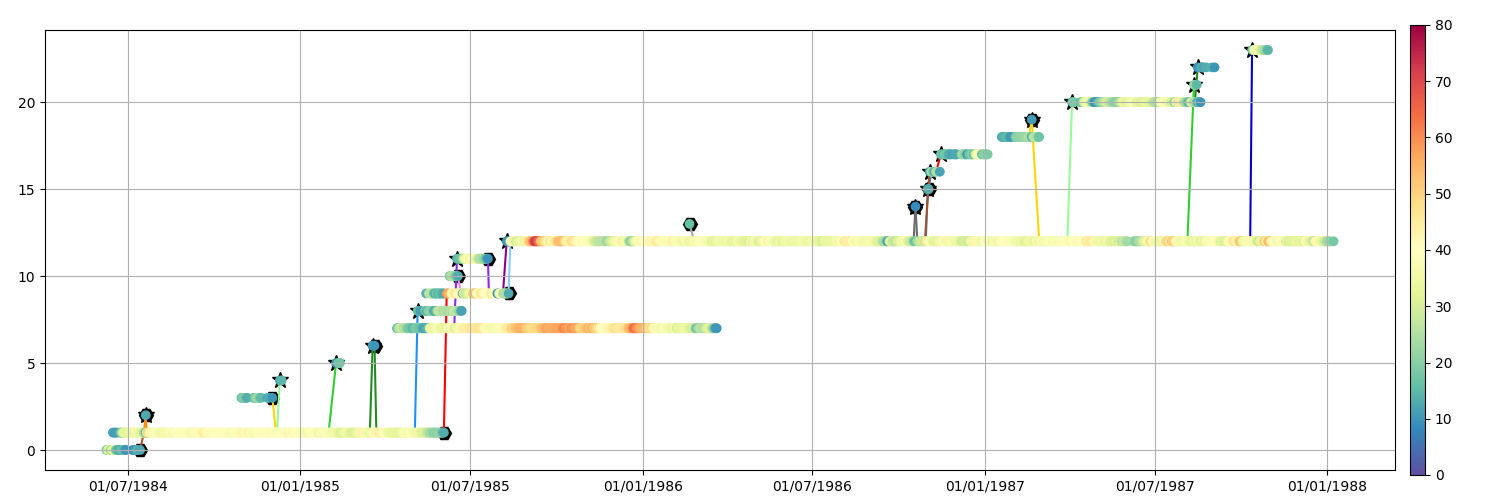
a/b
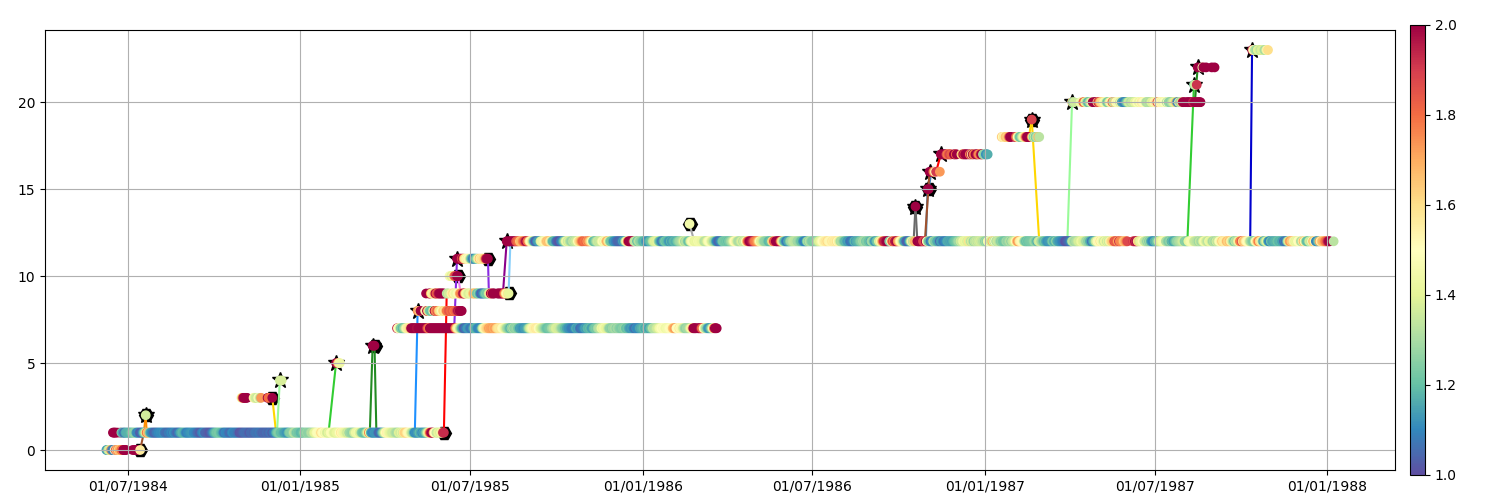
Total running time of the script: ( 0 minutes 29.232 seconds)